“Garbage in, garbage out” has never sounded so data-driven, especially when it comes to AI training data sources. If the data fed to the AI is flawed or biased, the results will reflect that.
When we talk about AI training data sources, we’re diving into where AI gets its knowledge from. The quality of these sources is critical because ,just like any student, AI needs good data to learn from. Without proper AI training data sources, AI’s understanding of the world might be flawed, leading to incorrect or biased results. So, whether it’s public datasets, medical records, or global collaborations, understanding AI training data sources is essential for building smart and ethical AI systems.
Now, if AI is like a digital child prodigy, then data is its daily reading material. But here’s the catch: if you feed it junk, you’ll raise a genius who can confidently say very wrong things. Enter the legendary phrase: “Garbage in, garbage out.” No matter how shiny the AI, if the data is trash, the output will stink too.
So… where does this magical, mysterious, and potentially messy data come from?
The Sources of AI’s Brainpower: Where Data Comes From
Training data doesn’t just fall from the sky (though it does sometimes come from satellites). Here’s where most of it originates:
A. Public and Open-Source Data
This is the AI buffet table—tons of free and available stuff from all over the internet.
Common sources include:
-
Wikipedia (yup, AI also gets stuck reading long entries about 18th-century warships)
-
Common Crawl (a massive scrape of the internet)
-
Open datasets for NLP and computer vision, like SQuAD, COCO, or ImageNet
Pros: Free, huge, and varied.
Cons: Not always clean or accurate. Biases, outdated info, and noise lurk around every digital corner.
B. Anonymized Medical Records and Clinical Trials
In healthcare, AI gets its lessons from:
-
EHRs (Electronic Health Records), carefully anonymized
-
Clinical trial data—patient results, lab findings, CT scans, and more
-
Research datasets like MIMIC-III (ICU data, not mixtapes)
Regulations like HIPAA (U.S.) and GDPR (Europe) ensure patient privacy. All personally identifiable info is scrubbed before AI touches it.
Pros: Rich, valuable, and can save lives.
Cons: Hard to access, tightly regulated, ethically sensitive.
C. Global Collaborations & Shared Datasets
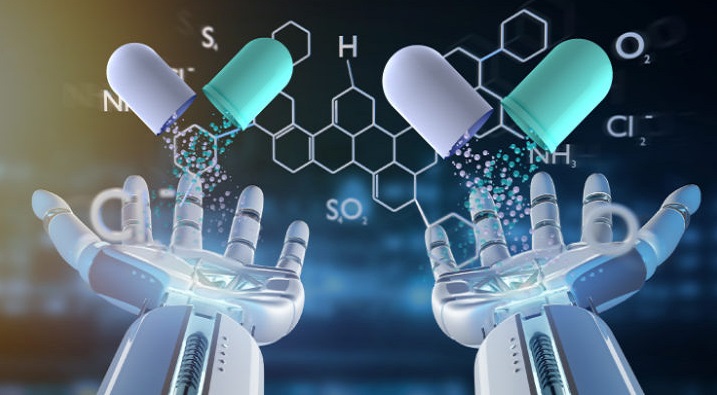
When big minds across countries unite, the data flows like an international science fair.
Examples include:
-
The Human Genome Project
-
OpenAI partnerships with global labs
-
AI consortia working on medicine, climate science, and safety
Pros: Diverse data that reflects a wider human experience.
Cons: Regulatory mismatches and cultural bias risks.
D. User Interactions & Online Behavior
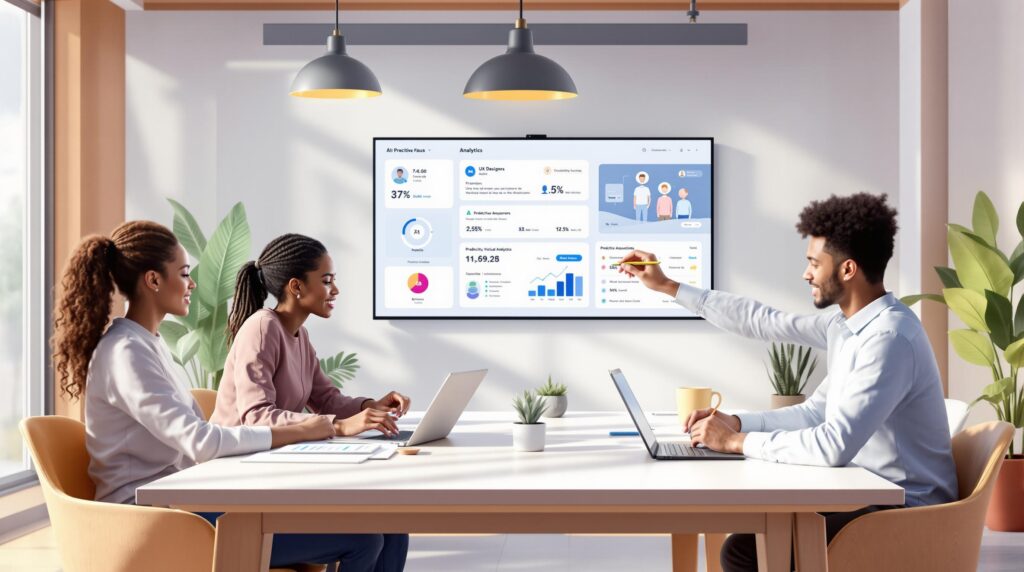
Yep, AI can learn from you—but only if you’ve given permission. Think:
-
Chat histories (like this convo!)
-
Product reviews
-
Clicks, scrolls, and swipes
-
App feedback
Data is usually anonymized and aggregated. Your private business stays private (if everyone plays fair).
⚠️ The Big Issue: Not All Data Is a Good Teacher
Here’s where it gets spicy. Even the smartest AI model can flop miserably if it’s trained on low-quality data.
Like we said earlier: garbage in, garbage out. Let’s break that down:
1. Biased Data
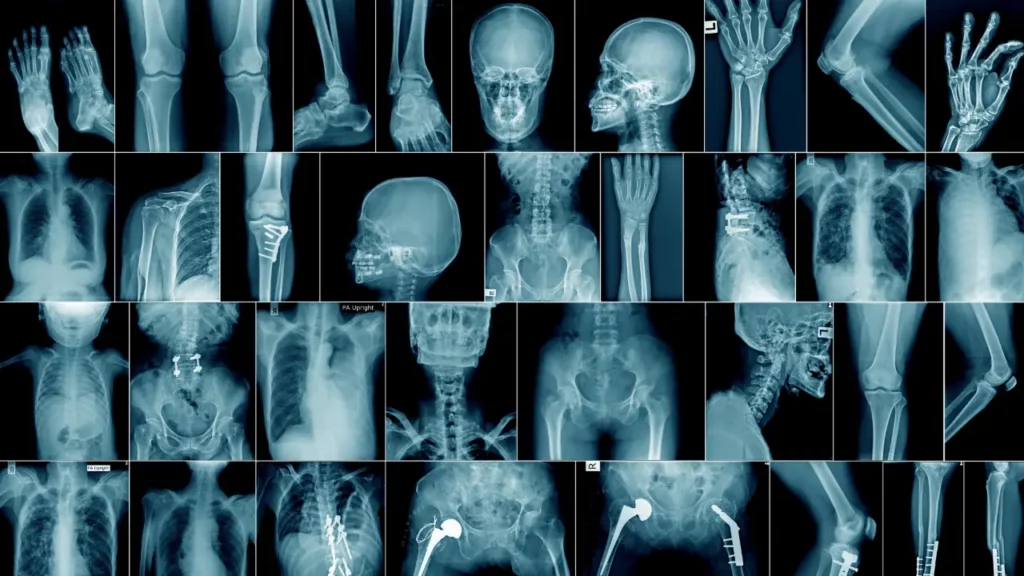
Say you train a medical AI using only data from middle-aged white males. That model might bomb when diagnosing someone who doesn’t fit that exact profile.
Bias can be subtle and deadly, especially in areas like healthcare or law enforcement.
2. Noisy Data
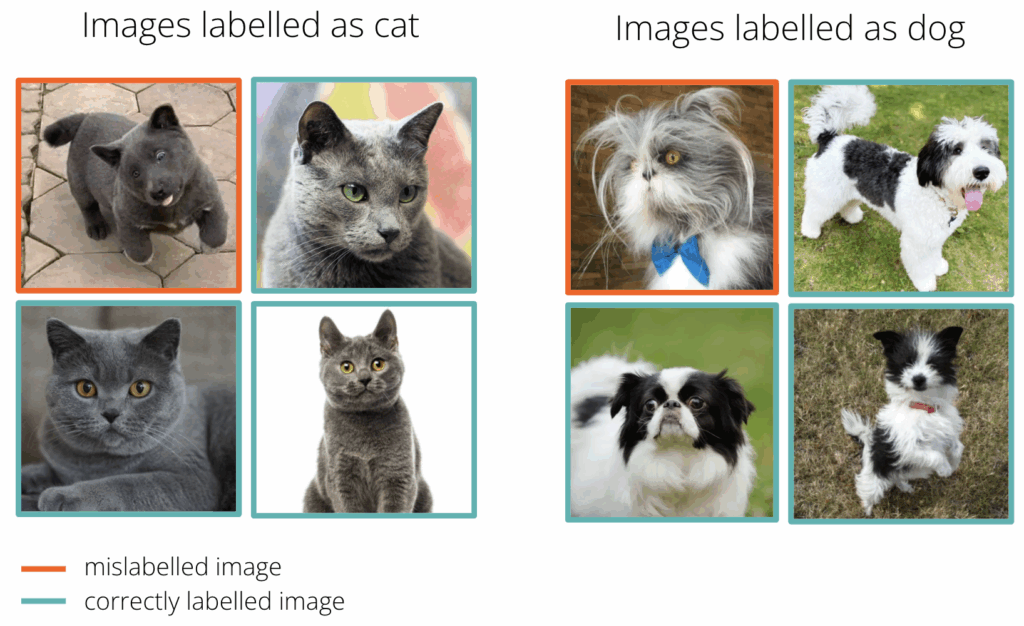
Imagine learning history from a textbook full of typos and fake facts. That’s what noisy data does to AI—it confuses the heck out of it.
Common culprits:
-
Spelling errors
-
Duplicate entries
-
Inconsistent formats
3. Lack of Representation
Training a language model with 90% English data when you’re trying to serve a global audience? Bad idea. The result? Great in English, awkward in Swahili.
4. Mislabelled or Poorly Annotated Data
In supervised learning, labels are life. If you tag a cat picture as “dog,” don’t be surprised when your AI thinks a lion is a chihuahua.
Fixing the Data Problem: Making It Clean, Fair, and Ethical
So, how do AI developers turn that data chaos into something useful?
Common Techniques:
-
Data preprocessing: Cleaning out typos, duplicates, and weird outliers
-
Expert labeling: Getting specialists (like doctors) to annotate images or text properly
-
Data augmentation: Expanding datasets by adding variation (e.g, rotating images, changing lighting, etc.)
-
Bias audits: Measuring and reducing unfair leanings in the data
-
Privacy techniques: Like differential privacy and federated learning, which help train models without ever seeing raw user data
Real-World Examples: What This Looks Like in Practice
A. AI for Medical Diagnosis (Radiology Edition)
Some models are trained on thousands of lung CT scans gathered from hospitals across Europe and Asia.
Challenge: Imaging standards vary from one country to another.
Solution: Calibrate the model with local data and retrain as needed.
B. Multilingual Chatbots
Imagine a chatbot trained on Wikipedia articles, news headlines, and community forums in over 50 languages.
Problem: Lesser-known languages get less data love.
Solution: Crowdsourced contributions from local communities—yes, the people help teach the bot!
Final Take: It’s Not About More Data—It’s About Better Data
You could have an AI model with billions of parameters and cutting-edge architecture, but if it’s fed junk from unreliable AI training data sources, it’ll spit out junk.
Think of data like the foundation of a skyscraper. If the concrete’s weak, that 100-story building’s gonna tumble.
That’s why “garbage in, garbage out” isn’t just a snappy saying—it’s a warning. The quality, diversity, and ethical sourcing of data directly shape how smart (or problematic) AI will be.
FAQ: AI Data Sources, Demystified
Frequently Asked Questions (FAQ)
1. Can data from social media be used for AI training?
Yes, it can, as long as the data is anonymized and follows privacy guidelines.
2. Is medical data always protected?
Yes, it must be de-identified and usually goes through ethical approval before use.
3. Can AI learn from data without labels?
Definitely! AI can learn through unsupervised or self-supervised learning methods.
4. Which countries are the most active in sharing data?
The US, European Union, and some Asian countries like Japan and South Korea are major players.
5. Is it possible to create AI without data?
Nope, AI needs data to function. It’s like the fuel that powers everything.
If you’re interested in learning more about how AI is revolutionizing healthcare, check out our article on AI in Healthcare Diagnostics: Adoption and Future Prospects.